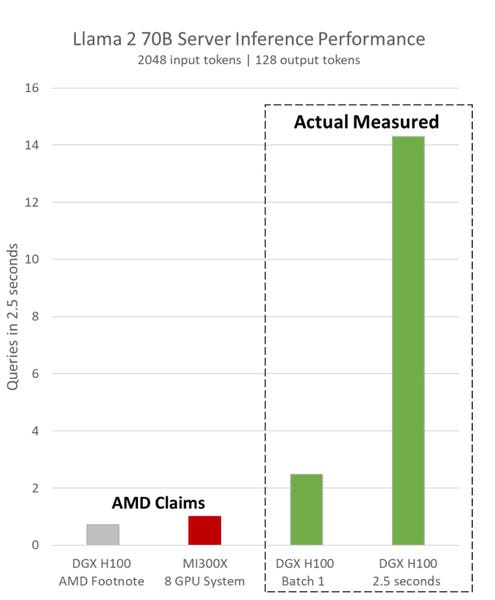
Au lancement du MI300, AMD a affirmé qu’il avait une performance significativement meilleure que Nvidia.
At the MI300 launch, AMD claimed it had significantly better performance than Nvidia. While the AMD chip does look good, and will probably run most AI just fine out of the box, the company did not use the fastest Nvidia software. The difference is enormous.
At a recent launch event, AMD talked about the inference performance of the H100 GPU compared to that of its MI300X chip. The results shared did not use optimized software, and the H100, if benchmarked properly, is 2x faster at a batch size of 1.
Nvidia has just released a blog that counters AMD’s claim that its latest chip, the MI300X, is 40-60 % faster in latency and throughput than Nvidia in inference processing for generative AI. Here is one of AMD’s slides at the MI300 launch event, which we covered here.
Below is from Nvidia’s counterclaim. While this sort of tit-for-tat isn’t what anyone wants to hear, it is massively relevant this time; all the press and analyst reporting I’ve seen echo AMD’s claims, which are inaccurate and misleading.
The latest results, run on software available long before AMD prepared their presentation, doubled the performance claimed by AMD. And with batching for the 2.5-second latency AMD used, a standard in the industry, Nvidia beats the MI300 by an astonishing 14-fold.
How could this happen?
It is simple. AMD did not use Nvidia’s software, which is optimized to improve performance on Nvidia hardware. “Though TensorRT-LLM is freely available on GitHub, recent comparisons by AMD used alternative software that does not yet support Hopper’s Transformer Engine and is missing these optimizations,” said the Nvidia blog post. Additionally, AMD did not take advantage of the TensorRT-LLM software that Nvidia released in September, doubling the inference performance on LLMs, nor the Triton inference engine. No TensorRT-LLM + no Transformer Engine + No Triton = non-optimal performance.
Since AMD has no equivalent software, they probably thought this was a better apples-t0-apples metric. These chips are expensive; I doubt anyone would not use the Nvidia software for production AI. It is free. “As LLM inference continues to grow in complexity, maximizing GPU performance on larger, increasingly sophisticated models using the latest inference software is critical to reducing cost and broadening adoption,” said Nvidia’s blog post.
What does this mean?
First, you can calm down if you are invested in Nvidia (stock or hardware). Nvidia remains the GPU leader. As Barron’s previously reported, “Investors Don’t Need to Worry.” And that was published before this latest news.
Second, if you are interested in the MI300X, we are not saying the new GPU is a bad AI platform. It appears to be the third fastest AI chip, behind Cerebras’ massive WSE CS2 (for which there are no benchmarks) and the Nvidia H100. And that is probably good enough for those seeking a more available GPU that should be reasonably priced (whatever that means; AMD did not release pricing).
The AI hardware market is moving extremely fast, and the H100 will soon become old news. The H200 is coming more quickly than AMD probably hopes. We note that the MI300 FLOP specs are indeed better than Nvidia H100, and the MI300 also has more HBM memory. But it takes optimized software to make any AI chip sing and translate all those flops and bytes into customer value. The AMD ROCm software has made significant progress, but AMD still has much to do.
« AI is moving fast. NVIDIA’s CUDA ecosystem enables us to quickly and continuously optimize our stack. We look forward to continuing to improve AI performance with every update of our software,” said Nvidia.
Conclusions
While all this may seem like a tempest in a teapot to the uninitiated, all silicon vendors should work carefully to ensure accurate performance claims with actual data (not just normalized bar charts) and provide all the details necessary to reproduce those results. Handicapping a competitor’s platform by not using the vendor’s software isn’t okay. That’s why MLCommons has published peer-reviewed MLPerf inference and training performance benchmarks every three months for several years.
Despite the kerfuffle, we stand by our earlier comments that AMD will sell every MI300 it can produce next year.
We asked AMD for a response and did not hear back.
When I asked Mark Papermaster, AMD CTO, if his company planned to run these benchmarks, Mr. Papermaster said they would publish MLPerf, but did not say when. We expect AMD will address the need for optimizations before they publish, and we can’t wait!